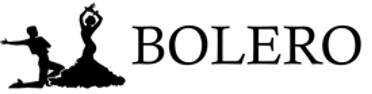
-
StatusOngoing
-
Status date2025-05-01
-
Activity Code1B.138
The project identifies, explores, and implements onboard continual machine learning techniques to enhance reliability and data throughput of communication satellites.
The first objective is to identify 3 most promising use cases and applications of continual learning (CL), together with at least two most promising hardware platforms.
The second objective is to implement different CL techniques in the selected scenarios and assess their performance and feasibility for onboard deployment using the selected hardware platforms. The assessment includes the analysis of advantages and trade-offs of CL in comparison to traditional offline machine learning approaches, and the comparative analysis of hardware platforms for CL.
The final goal of the project is to identify a state-of-the-art, potential gaps, and future roadmap for CL in satellite communication systems.
The main challenge is related to the limited resources and limited support for continual machine learning mechanisms in existing onboard processing units, e.g., not all operations and layers are supported and model parameters cannot be updated without hardware-specific recompilation. Therefore, common CL approaches are not straightforward to implement on board. Additionally, CL techniques come with the stability-plasticity trade-offs and the need of continuous validation and monitoring.
The project offers a complete software and hardware pipeline to implement 3 different continual machine learning approaches (i.e., class-incremental, domain-incremental, and task-incremental) in 3 different application for communication satellites. The comparative analysis helps to identify which approach and hardware platform is best suited for different CL scenarios. The project establishes the foundation for future development of CL in SatCom systems.
- Structured and informed report from selecting 3 most promising applications of CL in communication satellites and 2 hardware platforms
- Code for running a complete onboard CL process for both hardware platforms
- Report containing technology gaps and future roadmap for CL in SatCom systems
The 3 CL applications identified in the project are implemented for two hardware platforms of very different architectures (KP Labs Leopard DPU and BrainChip Akida neuromorphic computer). For each application and platform, there is a complete CL pipeline architecture proposed from data preprocessing to onboard continual learning.
The 3 most promising applications of continual machine learning in communication satellites have been identified, i.e., domain-incremental beam hopping optimization, task-incremental inter-satellite links routing, and class-incremental telemetry anomaly classification.
For each application, a state-of-the-art CL approach has been implemented for two diverse hardware platforms identified as the most promising ones for CL (KP Labs Leopard DPU and BrainChip Akida neuromorphic computer). The performance of each CL approach has been assessed and main technology gaps have been identified.