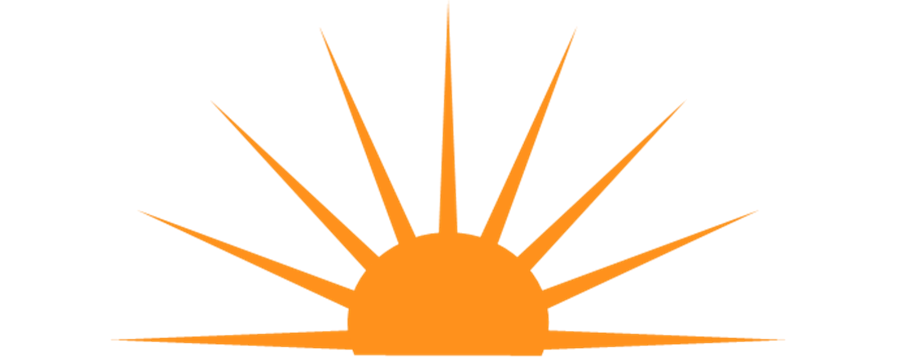
-
StatusCompleted
-
Status date2023-09-25
-
Activity Code3A.108
The DAWN project develops and implements techniques and algorithms that allow automated network control and management based on
-
network-generated information – data-driven networking –,
-
users' data – context-awareness –, and
-
measured and predicted performance of the network.
In addition to validating the performance of the algorithms through simulation DAWN also validates the proposed data driven techniques in the integrated 5G satellite-terrestrial test bed of SATis5.
The work programme of DAWN focuses on addressing systematically the following challenges. Firstly, meaningful use cases need to be selected and a reference architecture drawn up. Next, key performance indicators need to be defined, alongside the detailed placement of functionality and the analysis of AI/ML based algorithms. A methodology to assess the AI/ML solutions needs to be devised. Finally, the implementation needs to be tested and validated in a large scale testbed and through over-the-air demonstrations.
Regarding automation, resource federation and network control the DAWN approach relies on existing APIs as much as possible to facilitate smoother acceptance by the commercial market. However, when needed these APIs will be extended with additional functionalities.
As a final challenge, we plan to use DAWN results to steer standardization.
DAWN is expected to provide valuable insight regarding which performance aspects of networks can be addressed using machine learning and artificial intelligence techniques, identify candidate algorithms and approaches and provide an initial assessment of the scale of improvement that can be achieved using such techniques. All this verified in a large scale over the air testbed.
The DAWN project develops and implements techniques and algorithms that allow automated network control and management based on
-
network-generated information – data-driven networking –,
-
users' data – context-awareness –, and
-
measured and predicted performance of the network.
Regarding automation, resource federation and network control the DAWN approach relies on existing APIs as much as possible to facilitate smoother acceptance by the commercial market. However, when needed these APIs will be extended with additional functionalities.
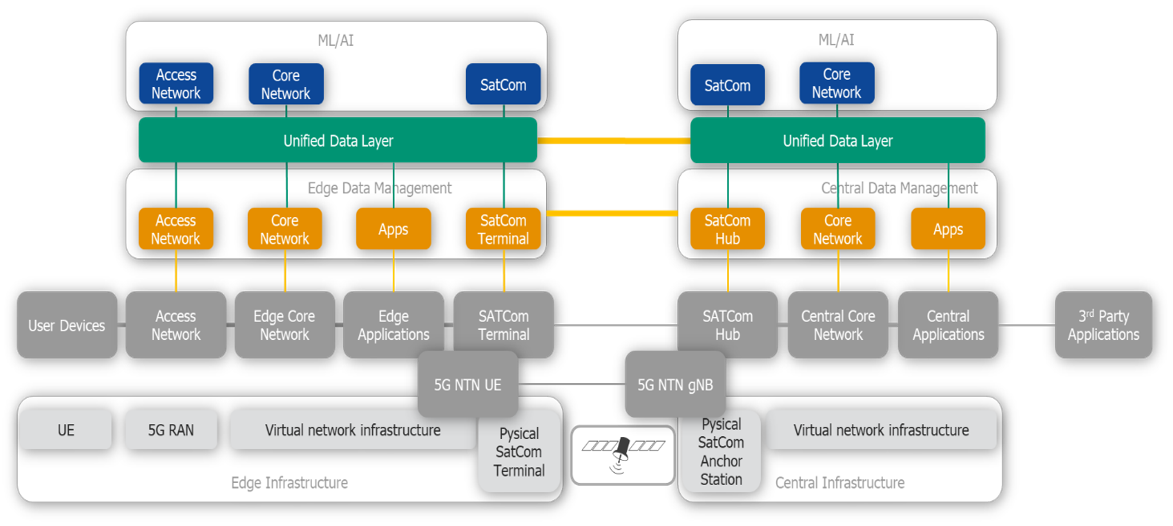
The DAWN work programme is planned to last 15 months and is structured in two phases. The first phase is planned for 6 months concluding with a design review. The second phase is planned to run for 9 months with a final review at the end.
The project was completed at the end of 2022.
DAWN has demonstrated the data layer integration and the functional interoperability of the test-bed prepared. The automation capabilities of the end-to-end system were demonstrated in terms of actuation based on the insight gauged from the monitored data.
The experiments have proven the fault detection to be practical, especially with the machine learning front-end prepared for it. The total delay in detecting a faulty condition was about 6 seconds, which makes it fast enough for corrective actions such as the topology switch and the swap of the core, which took several minutes.
The main achievements of DAWN are twofold:
-
The reinforcement learning performed in DAWN relied on using actual HW in contrast to the vast majority of research where reinforcement learning relies on using a simulator / simulated environment. The value of reinforcement learning results obtained using a simulator / simulated environment are more limited and questionable, therefore the approach taken in DAWN to use actual HW is exemplary.
-
Application of over-the-air tests to verify the in-lab results. In DAWN the test results obtained in a controlled laboratory environment were very importantly verified through over-the-air tests, further increasing the value and applicability of the results.