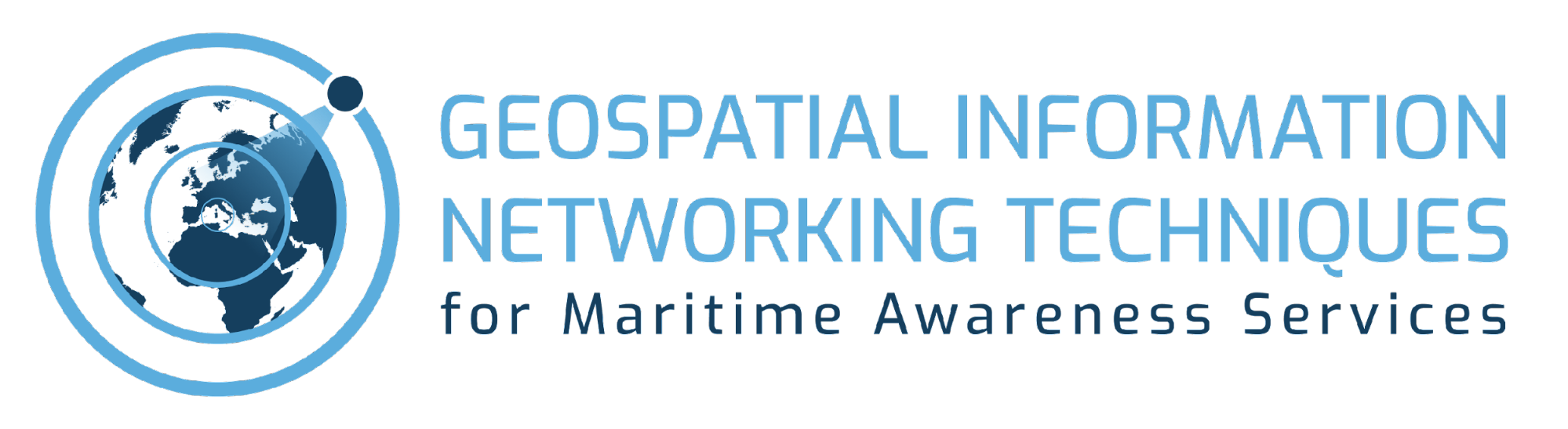
-
StatusOngoing
-
Status date2023-07-17
-
Activity CodeAT-4S -3A.
The automatic identification system (AIS) is essential to maritime traffic situation awareness. AIS tracks vessels' movements and is invaluable for detecting and monitoring suspicious activity. However, AIS can be switched off accidentally or deliberately to hide suspicious activities. These ships put marine traffic safety at risk.
Detecting dark vessels is more than having no AIS messages or observing when an AIS message is not received beyond a specific time interval. The reason is that AIS message transmission is affected by many factors, such as distance from receivers or base stations, weather and environmental conditions, and proximity to ports. Furthermore, AIS data lack information about the vessels' real-time environment and their reaction to adverse environments.
This project’s objective is to build a set of deep learning models supported by the integration of a diverse set of data sources which will allow maritime safety and security stakeholders to accurately:
-
Detect dark ships
-
Monitor the activities of the located dark ship
-
Locate the identified dark vessel
-
Support Search and Rescue activities
The key challenges for the provision of the Maritime Awareness Service System are:
-
Acquisition of suitable amounts of satellite data for machine learning model training purposes. The usage of multiple satellite vendors with different capabilities and external interfaces is required and post processing to have a baseline standard of imagery for training is required within the system.
-
Accuracy and availability of RF data. Since RF is a new and emerging technology additional research into its capabilities and selection of RF providers is required within the project.
-
Availability of ground truth in relation to dark activity of vessels. By nature the identification of dark activity has historical being difficult and is required for model training. Simulation of dark vessel activities will be used to increase the data set for ML modelling purposes.
-
Availability of historical archive of global AIS data for ML training.
The core product benefits and value relative to existing competing systems are:
-
Enhance the ability for reliable ship identification when AIS systems are turned off or disabled. The target is to detect at least 50% of dark vessels activities in selected regions of interest.
-
Enhance reliable ship location when AIS tracking not available. The target is to predict the track and location of the vessel correctly at least 80% of the time.
-
Enhance reliable ship identification. The target is to detect and identify at least 50% of dark ships activities in region of interest.
-
Compared to AIS-only, the target improvement in the detection is the one order of magnitude.
The Maritime Awareness Service main feature is to improve the current state-of-the-art in the domain of vessel detection and identification by fusing the data from multiple sources and through the implementation of ML models provide new ways of processing, fusing and analysing multiple data streams. This approach improves the quality of vessel detections and analytical capabilities for the maritime domain.
The key end user features are:
-
Applying the data mining and deep learning techniques to increase the accuracy of vessel detection
-
Applying the data mining and deep learning techniques to increase the accuracy of vessel identification
-
Forecasting the future tracks of the vessels and utilizing the forecasts to enhance the tracking of dark activity of non-cooperative vessels.
-
Support both insurance compliance officers, marine security and search and rescue end users through Maritime Awareness Service SAAS web application.
The following diagram provides a high-level overview of the three-layer architecture consisting of:
-
Presentation Layer
-
Application Layer
-
Data Layer
which is the basis for the Maritime Awareness Service demonstrator system.
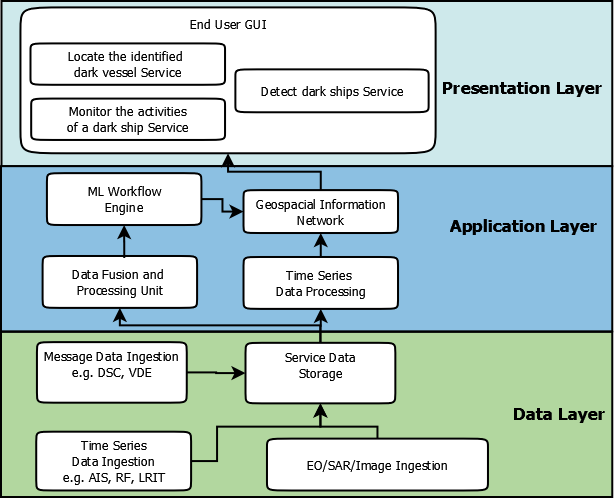
The key functional components are:
-
Web portal,
-
Imagery serving,
-
Workflow coordinator,
-
Data storage,
- Feed fetcher components:
- Copernicus fetcher for Sentinel-1 SAR and Sentinel-2 EO,
- SAR tasker and fetcher,
- EO tasker and fetcher,
- AIS fetcher,
- RF fetcher
- Vessel detection from imagery components:
- Sentinel-2 EO vessel detection component,
- Sentinel-1 SAR vessel detection component,
- Commercial EO vessel detection component,
- Commercial SAR vessel detection component
- Processors for tabular data sources:
- AIS processor,
- RF processor
- Matching engine
The project is scheduled to be completed over a 21 month timeframe with the KO taking place on the 13th March 2023.
The primary milestones and phases for the project are as follows:
-
KO – March 2023
-
Preliminary Design Review (PDR) – February 2024
-
Acceptance Review (AR) –July 2024
-
Final Review (FR) – November 2024
-
Final Presentation (FP) – December 2024
The project was successfully kicked off on the 13th March 2023 with the initial focus on selection of the high level use cases which form the basis for the system services.
To support the use case definition and refinement from the initially proposed selection within the project proposal, two workshops were held. The first workshop held at the Lloyds building London attended by key marine underwriters and compliance experts focused on the needs for dark vessel and identifying potential illegal activities. The second workshop was held in Maynooth University Ireland was attended by Naval Services, Coast Guards, Custom and offshore energy experts stakeholders.
The workshops clearly provided the core set of use cases and user requirements as the baseline for the Maritime Awareness Service. Based on end user needs, technical prototyping and the services technical baseline have been defined.
For the PDR milestone the detailed system requirements and system architecture for the Maritime Awareness Service where defined in addition to the external ICD and the approach for system testing and verification. The design detailed the components for the different data sources ingestion, covering the wide range of sources and types, to data fusion, application of ML, vessel detection and matching engines. The information is then visualised through a web application mapping interface GUI.
The current phase involves the component development based on the detailed design and the initial component system testing and validation in preparation for the Acceptance Review milestone.
The project was successfully kicked off on the 13th March 2023 with the initial focus on selection of the high level use cases which form the basis for the system services.
To support the use case definition and refinement from the initially proposed selection within the project proposal, two workshops were held. The first workshop held at the Lloyds building London attended by key marine underwriters and compliance experts focused on the needs for dark vessel and identifying potential illegal activities. The second workshop was held in Maynooth University Ireland was attended by Naval Services, Coast Guards, Custom and offshore energy experts stakeholders.
The workshops clearly provided the core set of use cases and user requirements as the baseline for the Maritime Awareness Service. Based on end user needs, technical prototyping and the services technical baseline have been defined.
The activities about to start are to take the technical baselines and user requirements and defined the detailed system requirements and system architecture for the Maritime Awareness Service to be presented at the PDR milestone.