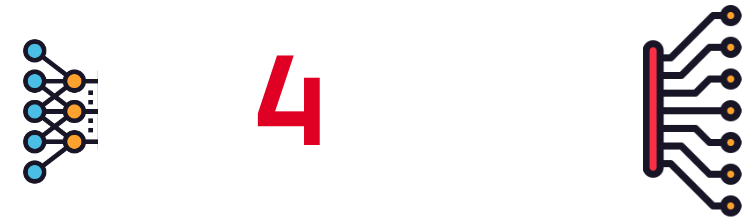
-
StatusOngoing
-
Status date2024-09-30
-
Activity Code6B.113
Design and development of software for network management in optical satellite constellation networks with machine learning techniques, benchmarked against solutions with classical network optimization techniques.
Improve already existing state-of-the art techniques for network optimization by using machine learning, bringing an improvement on link capacity utilisation of at least 50%, and demonstrated under a testbed capable of simulating a representative scenario running these new algorithms in the control plane.
This product will make use of new techniques bringing a flexible and highly adaptable control plane to optical constellations, useful in such an extremely dynamic and varying topology.
New machine learning techniques improving existing state-of-the-art techniques for network optimization and software testbed demonstrating the usage of these techniques in realistic optical communication scenarios.
To be confirmed during the preliminary design and critical design review phases of the project, the proposed demonstrator architecture would be based on an existing network simulation framework, i.e. NS3 or OMNEST++, extended by several modules already foreseen, such as configuration utilities for the scenario configuration, orbital propagation and visibility calculation, channel emulation and ISL management.
-
Definition of use cases and scenarios
-
System Requirements Review
-
Preliminary Design Review
-
Critical Design Review
-
Implementation and Verification
-
Acceptance
-
Final Review
The project was kicked-off on the 26th of July 2024, and it has started producing the first outputs for the definition of the different use cases and scenarios to be analysed in the project.