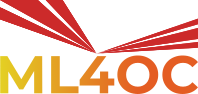
-
StatusCompleted
-
Status date2024-04-30
-
Activity Code6B.090
Optical Communication Systems (OCS) have successfully been used for high communication data rates, either between satellites or between satellites and optical ground stations. However, such activities are not well optimised, owing to the complexity of the system. To this end, this activity explored where Machine Learning (ML) can be applied to provide or enable such optimisation, through upgrading, improving, or simplifying OCS.
In ML4OC the focus was drawn onto improving the onboard laser communication terminals and the traffic routing in an optical network. These topics were studied in the view of ESA’s HydRON (High Throughput Optical Network) project to ensure network connectivity in orbit. To align with this, the project delivered demonstrations of ML models which showed their impact on the link acquisition control loop and network traffic routing.
The main activities and objectives of ML4OC project were:
-
Perform use case and requirement capture for ML-enabled OCS highlighting ML optimisation opportunities.
-
Develop and describe ML models along with test systems to be integrated into.
-
Demonstrate the benefits for the system by applying the ML solution instead of conventional methods.
The key technical challenge for this activity lay in the limited availability and suitability of datasets required for training ML algorithms targeting OCS use cases. Development of custom datasets and the systems to test them on was an intensive task and required many inputs from all parties involved. This posed challenges to the ability of the ML algorithms to show performance improvement over the baseline and to meet performance requirements.
Furthermore, there was difficulty in defining fully representative requirements to support the ML development due to limited detail on information as the HydRON project is in initial phases.
The outputs of this activity are machine learning models which improve spatial acquisition and network management in space to ground optical communication systems. This benefits future HydRON and OCS SatCom missions by improving overall availability and efficiency of optical links.
For spatial acquisition optimisation, ML techniques were used to optimise the detection of an optical uplink signal in the presence of poor camera sensor conditions such as dead/hot pixels and low SNR where the signal is at a similar level to the surrounding background noise. Different levels of poor SNR were tested, and the developed solution performed at a higher accuracy than a conventional detection algorithm.
Optimisation techniques were applied to the network management by identifying the status of neighbouring nodes and using the output to drive local control logic to locally re-route traffic around a degraded network link to maintain network throughput. The ML based traffic prediction and re-routing showed improvements over a conventional Software Defined Networking solution.
Supporting the spatial acquisition optimisation is a lightweight object detection model designed for real-time detection. The model takes in an image input and outputs the detected position of the uplink beacon. The chosen model showed versatility and robustness to different sensor data conditions. The high accuracy and high speed model enables detection to be maintained in poor sensor conditions.
For the network management, optimisation is implemented using localised healing functions, wherein degradation can be detected and recovered from by rerouting the traffic through nearby nodes. An ML model is embedded on each node in the network, wherein it monitors the traffic of the links on the node. ML prediction is then employed to predict the health of the links using latency and then the model enables a decision to be made on the best alternate local route for the network traffic. The ML assisted network nodes then communicate with one another so that the neighbouring nodes can also update their traffic flow tables to reroute the data until the performance of the original node is no longer degraded.
The system began with rationale, detailed descriptions and requirements of the optimisation opportunities and methods chosen for each use case. Then the ML models were developed and described for the use cases given (spatial acquisition and network management). For each use case, this involved the creation of the algorithms and the training datasets, and training of the algorithms. Following that, the algorithms were tested, validated, and verified using selected test scenarios. This involved the ML models being integrated into relevant test systems. For spatial acquisition this was an optical component test bench, and for network management this was a detailed network emulator. The results and performance of the algorithms were then analysed and compared against the conventional methods. Finally, the ML models were reviewed with the view of analysis of further opportunities.
Following the kick-off in February 2023, a requirements definition phase began. The first milestone was then the Requirements Review, which set the requirements for the remainder of the activity along with justification for proposed optimisation problems. The Mid-Term Review followed, covering description of the test system, breakdown of the ML implementation and documentation of verification and validation. The Design Review covered analysis and results comparison of the solutions. Finally, the Final Review closed the activity, and covered the final report and demonstrations which clearly outlined the advantages of the ML solution.
Currently, the project has officially kicked off, with the initial managerial deliverables such as the risk register, webpage contents and executive summary completed. The project has moved into the beginning stages of the first Work Package to clearly develop and specify requirements. This stage includes a workshop with ESA to ensure accurate capture of HydRON requirements.